
Technology
DEEP REINFORCEMENT LEARNING : EMPOWERING AUTONOMOUS SYSTEMS WITH AI
The field of machine learning has witnessed remarkable breakthroughs in recent years, particularly in the area of deep learning with applications such as image processing or natural language processing. However, supervised learning techniques have their limitations, especially when it comes to real-world scenarios where there are no predefined answers. This is where deep reinforcement learning (DRL), Delfox’s domain of expertise, comes into play, offering a new paradigm for training agents that can operate autonomously in complex and dynamic environments. By combining the power of deep neural networks with the trial-and-error learning approach of reinforcement learning, DRL has become a fast and effective way to engineer autonomous systems that can operate effectively in complex, dynamic environments.
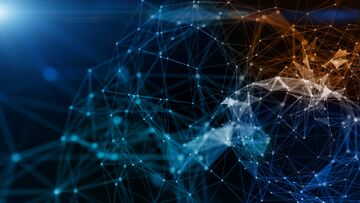
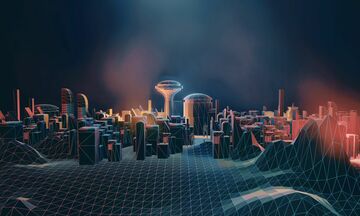
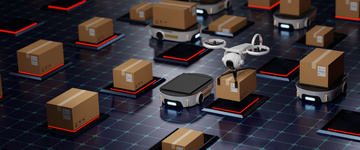